Read the article.
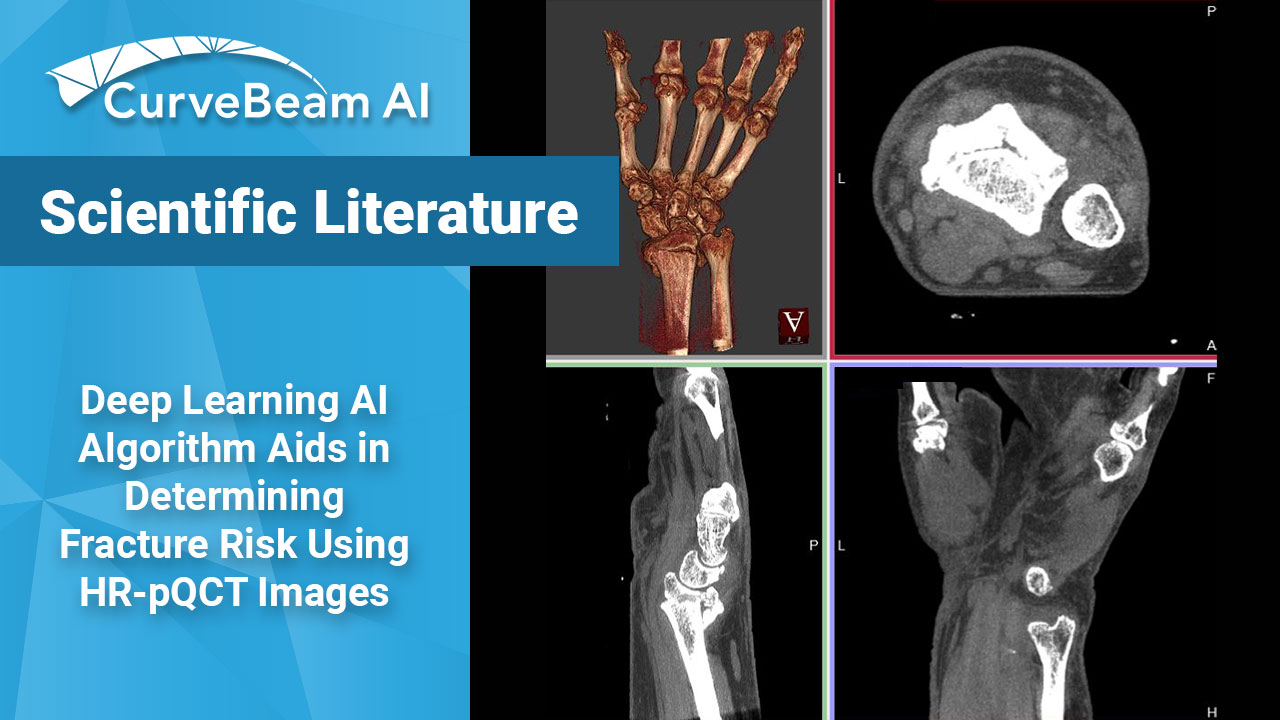
Deep Learning AI (DLAI) Algorithm Aids in Determining Fracture Risk Using High Resolution Peripheral Quantitative CT (HR-pQCT) Images
A recent publication in the online journal eLife has described the world’s first application of a deep learning AI (DLAI) algorithm to aid in determining fracture risk using images derived from high resolution peripheral quantitative CT (HR-pQCT) scans of the forearm.
Fragility fractures are a major health problem. Greater than 70% of women who have a fragility fracture are not identified using the current clinical definition of osteoporosis, and therefore remain unidentified and untreated.
The authors’ objective was to investigate whether this DLAI approach may identify women at risk of fracture before fracture. DLAI allows for investigation of bone’s multi-level hierarchical structure and soft tissue.
Cohorts of Postmenopausal women from Switzerland and France who considered representative of the general population were followed for a 5+ years. Three-dimensional images of the distal radius, ulna and soft tissue were acquired using HR-pQCT imaging. A Structural Fragility Score Artificial Intelligence (SFS-AI) algorithm was trained to distinguish between the 277 women who had fractures from the 1401 who remained fracture free during 5 years. SFS-AI was then tested in a validation cohort of 422 women. SFS-AI achieved a sensitivity of 60-68% and a specificity of 71%.
The eLife assessment of the study suggested that the work presented a valuable deep learning-based model for predicting fracture within the next five years, and that the evidence supporting the conclusion that the output of the SFS-AI can algorithm be used clinically to identify women at risk of fracture more effectively than with the current standard clinical approach is convincing.
Access the full study here.